🎧 Listen Now:
Welcome to the new Lead with AI podcast.
In this series, we speak to senior leaders responsible for rolling out AI in their organizations and uncover deeply valuable insights into what success looks like.
In today’s episode, we meet AJ Thomas, who previously led HR and Talent Acquisition at X, the Moonshot Factory, a sister company of Google. She’s now a fractional CHRO, coach, and investor, and in this episode, she shares:
- Why do we need to see AI as a colleague, a coworker, and not technology?
- What “the human in the loop” is and why it’s crucial to working with AI.
- Why understanding the history of AI, especially in the context of your organization, is a must to ensure you don’t just create problems faster.
- The importance of disciplined experimentation and how to create a “Design of Experiment” for your next AI project.
- And finally, why curiosity is the ultimate superpower for humans in the age of AI?
Key Insights from AJ Thomas
Here are the actionable key takeaways from the conversation:
1. Even in AI, the human is crucial.
As AJ said, “The human needs to stay in the loop.” The advent of AI doesn’t mean we blindly automate, that we set and forget. But we think about processes where humans and AI can seamlessly work together.
It’s also important to understand that AI is not a machine where you put in an amazing prompt and then blindly ship the outcome. You need to critically examine and validate AI’s work.
Train your teams on AI as a coworker, not as a tool that automates their work, and get them at ease with sound judgment.
2. Understand the History to Know the Future.
According to AJ, Generative AI is the fifth industrial revolution. It’s the current end point of a journey of 0s and 1s that started with big data, analytics, insights, machine learning, and NLP.
As leaders, we need to understand this evolution so that we know where we are in our experimentation, in selecting our systems, and in writing our policies. Without this understanding, you may create problems faster than you’re driving adoption.
If you haven’t caught up, dive a bit deeper into the history of what got us to Generative AI so that you can have a more informed opinion about what’s next.
And it’s not just the broad theory. You need to understand your organization’s data and AI journey. Therefore, partnering with your technology teams is crucial, especially on the people and talent side.
(Join Lead with AI to learn the history of AI + how to apply it today.)
3. Experiment, but in a disciplined way.
True experimentation with AI goes beyond surface-level engagement. AJ recommends following the ‘design of experiment’ approach that engineering teams practice.
Implement a structured experimentation process that includes defining clear hypotheses, designing experiments, and critically evaluating results to drive meaningful AI adoption.
And this doesn’t have to be a big, time-consuming project. Any team can ask AJ’s “one fun question”: "What needs to be true for this to be impactful for our business?"
Her example of letting AI analyze existing job descriptions and interview transcripts to find the best candidate is a great example.
Conduct thorough assessments of your business needs and potential outcomes before implementing AI solutions, ensuring alignment with your organizational goals.
4. And finally: Curiosity is the most important skill.
We’ve heard it from AJ: "The lesson here is to always be curious, ABC." As machines can already do everything, from baking burgers to generating images, our unique role will be to be curious about what else could be.
Continuous curiosity and learning are essential for successful AI integration.
Implement regular "curiosity sessions" or innovation workshops where team members can explore new AI technologies, share insights, and brainstorm potential applications for your organization.
And keep asking questions: Why did it do that? Can we make this better or faster?
Especially because no one has figured out AI yet, this can completely set you apart, and you can start tomorrow.
🔔 Available on:
Transcript:
Daan van Rossum: How do you see all of your background in talent experience, thinking about people in organizations, and AI itself? I think a lot of people are wondering, very practically, how do I get started with AI? What's your perspective on where space stands right now?
AJ Thomas: Yeah, I think for me, from the perspective I've had, I'm not an expert in this AI space, but I do know enough about it to be able to say that what I'm bringing to the table, I think, is the perspective of that human in the loop that is so important in the age of artificial intelligence and generative artificial intelligence.
There's no, I think, output without a really good input. And I think again that that piece of keeping humans in the loop—the work that I've done in the people and talent space—really connects all of those pieces together. We understand some of the concepts and frameworks that I think define a shared language for how we might adopt this type of technology in our workforces and in our teams. And personally and individually as well.
Daan van Rossum: I've actually been hearing that term quite a lot—humans in the loop. Can you maybe just share with the audience what they should be thinking about when they hear humans in the loop?
AJ Thomas: Humans are in the loop. So it's never just about automation. It's not something that you just set and forget. Especially when it comes to new technologies, it requires a deep amount of curiosity, and it requires a deep amount of making sure you have enough perspectives to make an informed decision around how you interact with them—basic principles of interaction in any sense.
And so I think this human in the loop that you're hearing about and that we're all, I think, talking about is really about reminding us. This is not just something where we're going to input a lot. It's coin-operated. Okay. It's going to do its thing. We're going to go into ChatGPT, for example, ask a really amazing prompt, take that thing, and then ship it.
(Check out our SuperPrompting Course for 10x Better Prompts)
We always have to be in the loop about that, reviewing it, looking at it, and having a discerning eye around what it actually is that it's come out with. So that we can validate that is the right of generative interaction that we have, and it can then create more capacity for us to actually think about things even further, treating it really more as a perspective that can help inform us as humans. I think that's the piece that we need to be thinking about when we think about humans in the loop.
Daan van Rossum: You're already talking about people looking at it very differently than a traditional piece of software. We're talking about having good judgment and knowing what kind of input you need to give for what kind of output.
What are some operating principles you would think about when it comes to releasing AI into your company? Because I'm sure your team was very AI, but there's a lot of people still trying to figure it out.
What have you learned from how you embrace AI with your team that other people could pick up?
AJ Thomas: I think that's a really great question. I think over the many iterations of teams that I've been in, and I hope you don't mind, I go into a little bit of a history lesson from the learning.
It's interesting that we are in the fifth industrial revolution. If you think about steam power being the first revolution, electricity, electronics, and hardware, and then AI robotics and big data, and now really about this generative piece of how you actually interact through remote access and all of these different pieces,.
If you even break that down when we think about AI, as leaders, I think it's really important to understand the history and context of where it has come from. In the 4th and 5th industrial revolutions alone, you see the transitions of them building upon each other, where it's really a journey of zeros and ones and zeros and one being data.
Again, this is my understanding from a lot of learning, but there's zeros and ones. And then those zeros and ones eventually became just that: that's big data—a lot of data. What do we do with that huge amount of data in the corporate space? It was huge from a security perspective to figure out what that was.
Once you had that big data, you had analytics and insights. Okay, now that we have all of this information, we have to make sense of it. We have to help drive decisions out of it, and a lot of your SaaS companies came out of that; enterprise SaaS companies came in and started. Hey, we can organize this for you. Here's a platform. Here's what it looks like.
The next iteration of that then became machine learning. So from the analytics and insights, we figured out, wow, now the data, we need to figure out how it can organize itself so that we can even get faster in the decision-making or whatever it is that we're pushing forward with what we're learning.
From the machine learning, then came the natural language processing work. That then inevitably turned into opening the floodgates for us—not only the computer interacting with itself in the data, but actual individuals and humans interacting with the computer itself. And that is the advent of the generative AI space.
And so I think again. I look at that chain, and I say, Okay, how many of us as leaders understand the evolution of that enough that we can say, Where are we in our experimentation? How mature are our systems and policies? Where are we in that?
Because you can apply a generative AI solution, but if your company's operating system is still just analytics and insights, you actually haven't set up the fundamentals to be able to experiment, and therefore you may be generating problems faster than actually trying to fix them and create opportunities for massive adoption of the technology, as you would want to imagine and use it for impact.
Daan van Rossum: That's a very interesting perspective. If we don't understand where we are in our journey and we just put a generative AI on something, it's actually not going to help us. It could actually hurt us. You're saying you could actually create problems even faster.
You do hear a lot of people say that this is just the time for people to experiment; just give them the tools. They will figure out the use cases. We saw that in a couple of the case studies.
So where is that balance? We've seen some data showing that employees are actually running faster than companies. So they are the whole BYO AI movement. People are bringing their own ChatGPT to work with all the risks that it has.
Where's the balance between taking that step back, understanding the journey, maybe from a broader technical perspective, and making sure you don't miss the boat as everyone else is already on it, Gen AI?
AJ Thomas: Yeah, absolutely. And I think that's the shift in perspective, right? Because it's not about missing the boat. It's actually understood that if you're on a boat, a plane, a car, or whatever it is, that's transporting you to this next phase.
What I'm seeing a lot of is leaders really partnering with their technology teams. Specifically on the people and talent side, I'm going to mention somebody that I admire in this space. His name is Arvind KC, and actually, his role is very unique. As a leader, he actually leads the corporate engineering team and the people team and has combined people and systems in his current CPO role at Roblox.
I think that is beautiful because his journey has been as a CIO at Palantir and a corporate leader at large companies like Meta and Google. And what's been really interesting in spending a lot of time with KC is really understanding that a lot of the systems that he knows he has to build with his teams have to come informed with some very basic fundamentals of the human condition, humanity.
And so I think I'm not anti-experimentation. I have come from nearly half a decade where all we thought about was experimentation. But the discipline of experimentation for us is really getting deep down into what you are trying to disprove.
Experimentation. I think what I've seen is that people use it as a badge to go do something. It's my VIP badge to get into the club and do my thing at the generative AI club. When actually experimentation is about saying, Let me get curious real quick around, what is this opportunity space? What is my hypothesis?
In engineering, you have this thing called the design of experiment. I had the opportunity to actually serve a residency within our rapid evaluation lab at the Moonshot Factory at X. And what was really great about that was that I worked alongside some of the most amazing radical technologists and scientists who taught me from a people perspective and then from a technical perspective that a DOE, or what they call it, the design of an experiment, is just as important as what you're experimenting on.
By the way, in experimentation, you have to go into that, not trying to prove it's right, but actually trying to prove it's wrong. And in being delighted and surprised that, oh, we were right about this,.
And so I think that's the nuance that, as leaders, we need to think about in this age of AI: are we experimenting to get into the space or are we truly experimenting to look at the opportunity ahead and to disprove our hypothesis? I think more than ever, leaders need to have the DNA of a scientist and the expertise of a technologist. And then they need to have the mindset of a people leader to be able to marry these things together and actually advance in this space.
Daan van Rossum: I was going to say that it sounds like you're truly approaching it as a scientist and you want to prove yourself wrong. You want to prove your hypothesis wrong. So you're really setting up the experiment with the objective of trying to disprove something. Now again, that may sound really interesting. It may also sound like a lot to a lot of people who will look at their calendars tomorrow and say, I have enough to do. That sounds like something you wouldn't do.
Is there something in there that you could practically apply no matter what kind of team you have, and maybe no matter what kind of workload you have? Obviously, for you guys, you knew this was something you had to make time for. You have to make room for it. It was probably in your DNA and in your whole mission to experiment a lot.
How do you scale that down to the point where it's approachable for anyone to apply?
AJ Thomas: One fun question. If you realize that there's something you want to either explore to disprove or make true, or whatever I think it's four words, what needs to be true for this to do this? Even if you're a busy executive and you're handling a problem or there's some sort of impasse that you just can't get around, if you're implementing a new policy around artificial intelligence and what functions can use that right in your organization, if you're a busy leader with back-to-back meetings and everyone's coming at you with a ton of inbound, you can ask a very simple yet impactful question. It's really interesting that we are leaning into this space on the legal side to use generative AI as an example.
What needs to be true for that to be impactful for our business?
Daan van Rossum: Then you're challenging people internally to say, I'm not saying you shouldn't try it, but I want you to try it with more purpose. I want you to try it with a crystal clear objective in mind so that we can actually look at that experiment and say, Did it work or not? Because obviously, endless experimentation sounds really interesting, but it may not lead anywhere, and like you said, it may just create problems faster.
AJ Thomas: Yeah. So, I'll talk about this, and I've said this many times before, right? We live in a world where, for the past couple of decades, credit goes to Simon Sinek, who really simplified this aspect of why, how, and what. Everyone knows how it starts with the why, then what's the how, and then what's the one you work backwards from there.
I would implore you to say that in this day and age, we don't forget “who" talks about mindset. Why talk about your purpose and why you're leaning into this thing? How does it talk about how you are going to get this thing done? What talks about what it is you're going to deliver?
We often miss the who in all of that. And the who in all of that is not about who you are to be doing this thing. It's actually who I am in my own mindset, and I think about this when it comes to the work of access, inclusion, and bias and diversity perspectives.
It can help you check your own bias, to say, who am I, as I'm leaning into asking this question of what needs to be true? Oh gosh, I'm representing a whole sort of point of view on this that I think I need to put on the table as a perspective to share with everyone else so that we can have a shared language around how we look at the problem.
What I see when executives, leaders, teams, and even individuals solve problems is that it's you, there’s me, and there’s the problem. And this is where you get into—well, you have a different perspective. I have a different perspective. Let's disagree and commit.
When actually I would implore us to say, okay, what needs to be true for us to have a shared view on the problem or opportunity space so that it becomes me and you against whatever this thing is. So it doesn't become a three-sided thing, but it becomes actually a very productive dialogue, not a tria-log where you're just chasing tail.
Daan van Rossum: And there's also another layer to that: where are the boundaries between the human employees and the AI employees, or the human team members and the AI team members? There seems to be a shifting perspective, like the moment people start experimenting, they also realize that a lot of what we thought we were uniquely good at, AI, can actually be done, not as the final product, but at least as that first draft or that first kind of idea. And where do I stop? And where does the AI start? And especially within the team context. So is the “who” also shifting in that equation?
AJ Thomas: Yeah, no, I love that question. Again, as somebody who just loves seeing the history of this thing, probably a lot of folks know the movie Hidden Figures, and it talks about the amazing women of color, these black women that went and really changed the trajectory of the United States going to space.
_____ by the name of Dorothy Vaughan is an amazing story here, Daan, because what she did was show the power of curiosity, right? The curiosity that she had. So, these big computers that were coming to NASA were going to eliminate all of the jobs of all of the admins and the computational mathematics that was happening in that space. And IBM came in with these big things.
And you know what she did? Instead of seeing that as something that's going to take my job, she got really curious, went to the library, learned the language in which that big computer was trained, which happened to be Fortran, really studied that, and then trained her team around the constructs of what that was.
Push came to shove. There was a moment where the machine actually malfunctioned. Everyone thought, Oh, they're going to take my job. It's going to be bananas. And guess what? She met the opportune moment with her team to say, as the executives were looking around at NASA and IBM, does anyone know how to actually work this thing?
She came in, and because she was curious and her team was curious, they invented a new capacity for them to be able to contribute to the impact of that transformation of technology in their workspace to accelerate that technology.
I think I share that story because, when you think about who is changing,. I think the mindsets need to change. AI is going to be a part of our team. We're hearing this term co-pilot. Co-pilot this. Co-pilot that. Co-pilot all the way. There are so many co-pilots; no one's going to be a pilot because everyone's a co-pilot, right?
When actually, I tend to be a little bit more of a first principles point of view on that, which is, I actually think about the technology as another perspective to be curious about. No one is going to replace the human aspect of what we bring to the table.
Curiosity. There's a reason why we need prompt engineers. We need them; they don't know how to be curious. So we have to be curious and prompt them. And so I think in your question, in the spirit of, is the who changing? I don't think we're there yet. And I would redefine that as what is the mindset that we have to think about it from a perspective, and how can we, with our human capability and capacity, be curious enough to then meet the moment when that does happen?
There are robots creating burgers for us right now. There are everyday robots that are cleaning things and moving things. There's autopilot in your car. But there's no amount of curiosity that will be replaced by that at this moment in time.
Daan van Rossum: The human quality that will always be there and will always be needed is curiosity. But that idea of getting more curious about what this could be versus what thread it poses or what part of my job I'm going to take away is obviously also important. You cannot expect the AI to be a good partner or a good co-pilot if you're not curious about what it can do.
What are some applications you're looking at with the most interest? What are some things that you're seeing that pique your curiosity?
AJ Thomas: Yeah. I think there's a couple of ones that are interesting to me: the application of the voice co-pilot, like everyone's into text right now. Oh, I'm going to text this. I'm going to put a bunch of data in something, and I'm going to have it realized.
I think there's a lot of power in this, and we've seen this with the Siris, the Alexas, and the Cortanas of the world, but I think there's another next stage to that, where there is this technology that I'm very excited about, where you're using a lot more voice to interact versus being on a screen to interact, so you can be more present.
(Check out our recommendations of the best AI websites here.)
I know there's nascent technology right now. Humane is doing some of that work. On our iPhones right now, we can do some of that work. I think ChatGPT in the 4.0 version, even in the 3.5 version, when it was released, had some of the voice capability.
So I think I'm really excited about leaning into that and the data that comes behind the tonality of voice and the regional geographical aspect of people's local dialects. And how does that become another source of big data to be able to analyze, create insights from, and learn from that will advance other pieces? And so, I think that is really interesting.
Imagine when you're in a meeting and you have something on your mind, whatever it is. Supernormal is doing some of these things right now, or Otter AI, these Fireflies notetakers. I'm excited to see how that actually embeds as a general part of something we interact with every day. Then I can sit down at the end of the day and say, Hey, how was my day? Not just how was this meeting, right?
Daan van Rossum: Yeah, I don't remember what happened today, but at least tell me.
AJ Thomas: Can you tell me what happened? And yeah, I don't know. I think there's a really interesting application for that. That's still a little bit nascent. As you can tell from the technology transformation, everything's a little bit scattered until people kind of start coalescing on different themes. I'm just really excited to see what kind of application that looks like in the real world. How might we test that?
Daan van Rossum: It may also be a lot more human. It's not that we don't text with people. That's obviously a big part of it. But I think why the movie Her spoke to so many people and really let people imagine what would happen is because it's so voice-based and voice is so personal, and it's so you connect way more with a voice than some command interface. So maybe another level on the Turing test in terms of voice.
AJ Thomas: Absolutely.
Daan van Rossum: What are some initiatives that you've rolled out to let people do guided experimentation, putting out some hypotheses? How could AI help us? And how did you get people? And again, this may be because you're running very different kinds of teams because you're working in super-tech companies, and you probably have the smartest people who do really have that tech background.
But even in those teams, do you still encounter some people who are anti-AI or who don't want to go along with new AI implementations? How did you manage that?
AJ Thomas: Of course. I think there's always a spectrum of that in the different teams that you're working on. The fear and creativity continuum when it comes to generative AI—it's really stinging. And again, AI has been around in the form of machine learning. Like I just said, there's autopilot in your car. That's a form of artificial intelligence to tell you when you need to break switch lanes, all of that good stuff.
And so I think in teams, like what I've seen, applying this very practically to hiring, applying this very practically to performance management, for example. A hypothesis that my team and I had previously was what needed to be true for us to improve the candidate experience and hiring manager experience, yet shorten the time for us to be able to have more meaningful, in-depth conversations.
That was like the design of the experiment—it needed to all be met. Candidate experience, employee experience, shortened time so that capacity of time could bleed into more deeper questions so that you actually have a way to look at the quality of the hire versus just the bullet point things on their resume on things that they've done. And I think that can apply to any organization.
So one of the experiments that we did was a regular intake meeting for hiring. And we noted all of the different pieces. We then took into account the requirements for the level of the role. And then we took the job description. And we took all of that information and put it into Gemini. And asked Gemini, which is Google's AI, to architect for us a series of questions that could help us check for these values, which we had in the organization.
We ran an A/B test on this. To say, okay. So, first of all, manager candidate, piece. It was really interesting because the information and the questions came back. We then took the hiring managers and the recruiters, and we said, Okay, let's now work at this and prioritize which skill sets.
Because this is what everybody wants: everything. And therefore, if you want everything, you get nothing. We know that in hiring, you can't always get what you want, but if you try, sometimes you might just get what you need. I think that's a Rolling Stones quote or song.
So what was really interesting is that we were actually able to align. We didn't set out to align the hiring team. We knew that was an assumption we wanted to get to an outcome, but because we did it this way, the outcome was that we came up with three theories that prioritized skill sets and expertise that we needed. And we then decided and voted on that as a team.
And the hiring manager intake and calibration meeting became much more in-depth because we had a shared language that we all co-created based on what we thought was important in the role and what we weren't regarding the candidates. Oh, we changed this, and we changed that, and we changed this, and we really went after some of the very key things. The other role we had took much longer, and we had to go through a third and fourth set of candidates because the job description and priorities kept changing.
So that's a very practical, very interesting A/B test. Implementing something very basic—the ingredients you already have in the kitchen, your job description, the transcript of your intake meeting, your leveling guide—put that in, spit some interview questions and top skills, go and see if you can vote on that as an interview team, run that test, and sprint.
We were able to hire this other candidate; I think it only took us like 20 days. Previously, it would take you three months or more. That's a very practical piece that we did on the talent side. I was very proud of the team that did that.
Daan van Rossum: I love that. That's great. I'm sure you did other experiments after, but what are some lessons, and why do you think it was so successful in the way that you set it up?
AJ Thomas: I think we were still learning. I wouldn't say we claimed it as a success because there was still a lot of automation. I think there's a lesson, like if you can impart one thing on this, I think the mindset is that, going back to the who, you really have to cultivate a culture of super learning in your team as leaders, especially with embedding technologies and transforming to digital, or whatever it is, whatever phase you're in in adopting technology. I think the lesson here is to always be curious, ABC. Always be curious.
Oh, interesting. Why? Why did it do that? How did we get to the point where we actually. So, within our team, we were asking questions, and we were delighted and surprised. But then we were like, Oh, could we make that faster? Oh, but then if we make it faster, is that actually good or is that bad?
And again, it doesn't mean that you have to wax and wane on this because sometimes, as a startup, you just have to get stuff done. I don't have time to debate this. I get it done together. Move forward. I absolutely get that. But it takes you maybe 30 to 45 seconds just to point, just to reset. So you're not on autopilot, to say, Oh, interesting. That went a lot faster. Was that actually good or bad? And how do we go?
Daan van Rossum: And having that dialogue around it. So it sounds like you set them up, you created the experiment, you did the design of the experiment, people go into it, and then you can reflect on it and analyze it. Most of that doesn't happen when people say, Oh, just go and experiment.
So I think you've really given people something in this episode. A very practical guide to, yeah, but what does good experimentation look like? So I think that's super helpful.
And I know that we're almost out of time. So I guess one other final thing that you would say is that if there's one thing that leaders, and again, we have listeners who have never done anything with AI and know that maybe they're even falling behind a little bit on their own colleagues and employees, what is something that anyone can do tomorrow to again get themselves, their teams, and their companies more AI savvy?
AJ Thomas: Yeah, I think understanding the context of how it has evolved generally, the journey, and how it has evolved in your organization is important. Get curious. And then also another C. Have the courage to ask the questions without blame or judgment on yourself, on your function, on your leaders, or on your organization. Everyone's trying to figure this out. No one has the silver bullet around this.
And I think the more curious we can get, the more super learning skills that we embed and encourage in organizations in our teams, the better we're going to be able to have a collective way in which this is applied.
It sounds like a very basic, first-principles kind of thing. And I would just implore us, in this age where we have so much more noise than the actual signal we need, to pay attention, be the person on the team, or encourage that person on the team to play the role, to ask the reset question. What are we optimizing for, and what needs to be true? Let's understand the context.
Daan van Rossum: That's fantastic. Because I do truly think there are so many people out there. Who is trying—maybe some imposter syndrome or something? I'm more senior. Therefore, I should know it. I think you just told it to everyone. We're all learning this at the same time. No one actually knows what this thing is.
We don't know what it will look like six months from today. So keep asking the questions, have the courage to ask and to be open and vulnerable about where you are, and then experiment together in this way. I love it. Thank you so much, AJ, for being on.
AJ Thomas: Thank you so much for the opportunity to share.
You Might Also Like …
Lead With AI

OpenAI Cofounder Ilya Sutskever: "We've Hit Peak Data." What's Next for AI?
Our latest articles
FlexOS helps you stay ahead in the future of work.

From “Smoosh” to a Better Hybrid Workplace – Liz Burow discusses key points of hybrid work in 2025

How To Get Started with AI in Recruiting
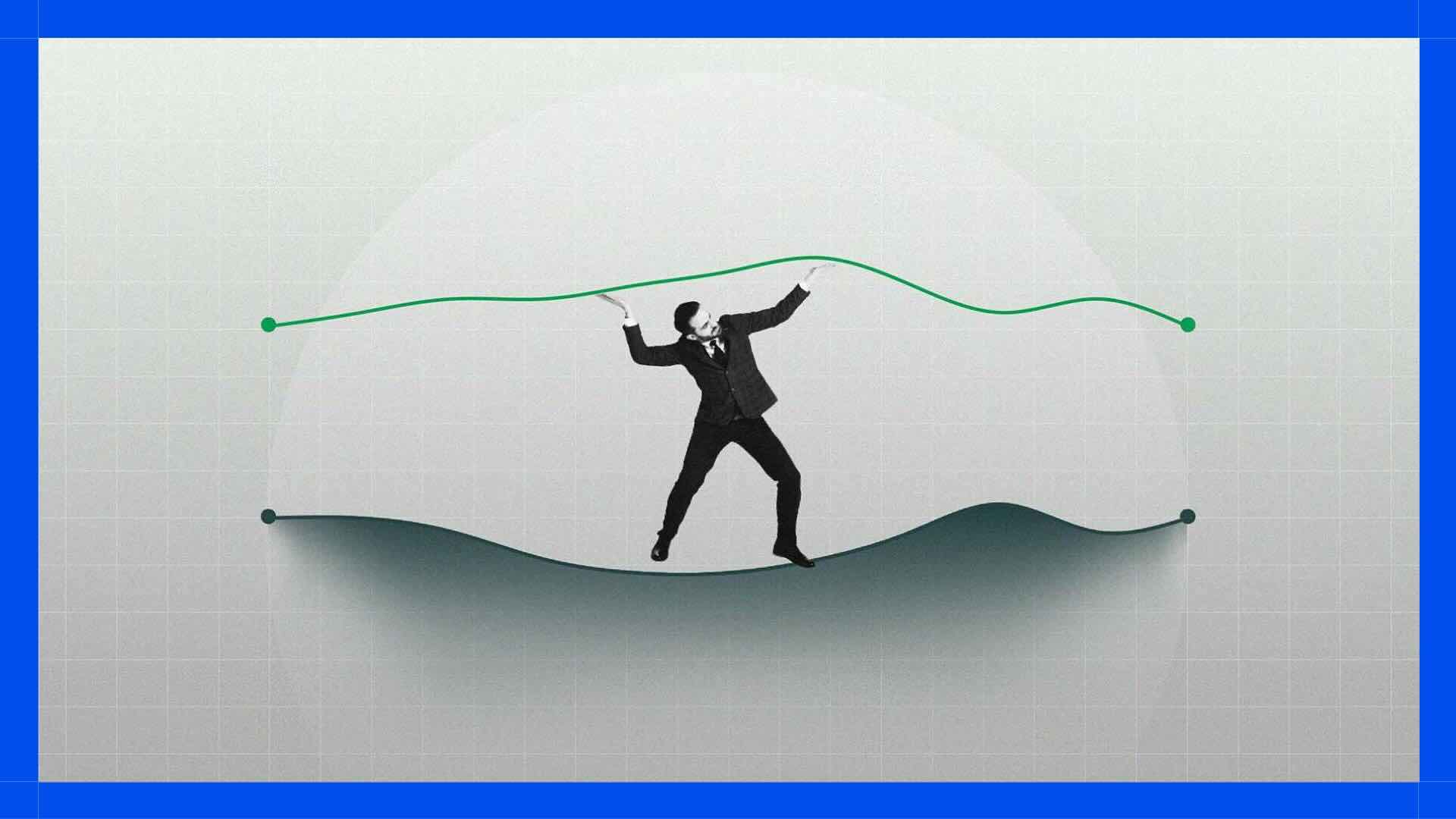
U.S. Employee Engagement Hits 10-Year Low
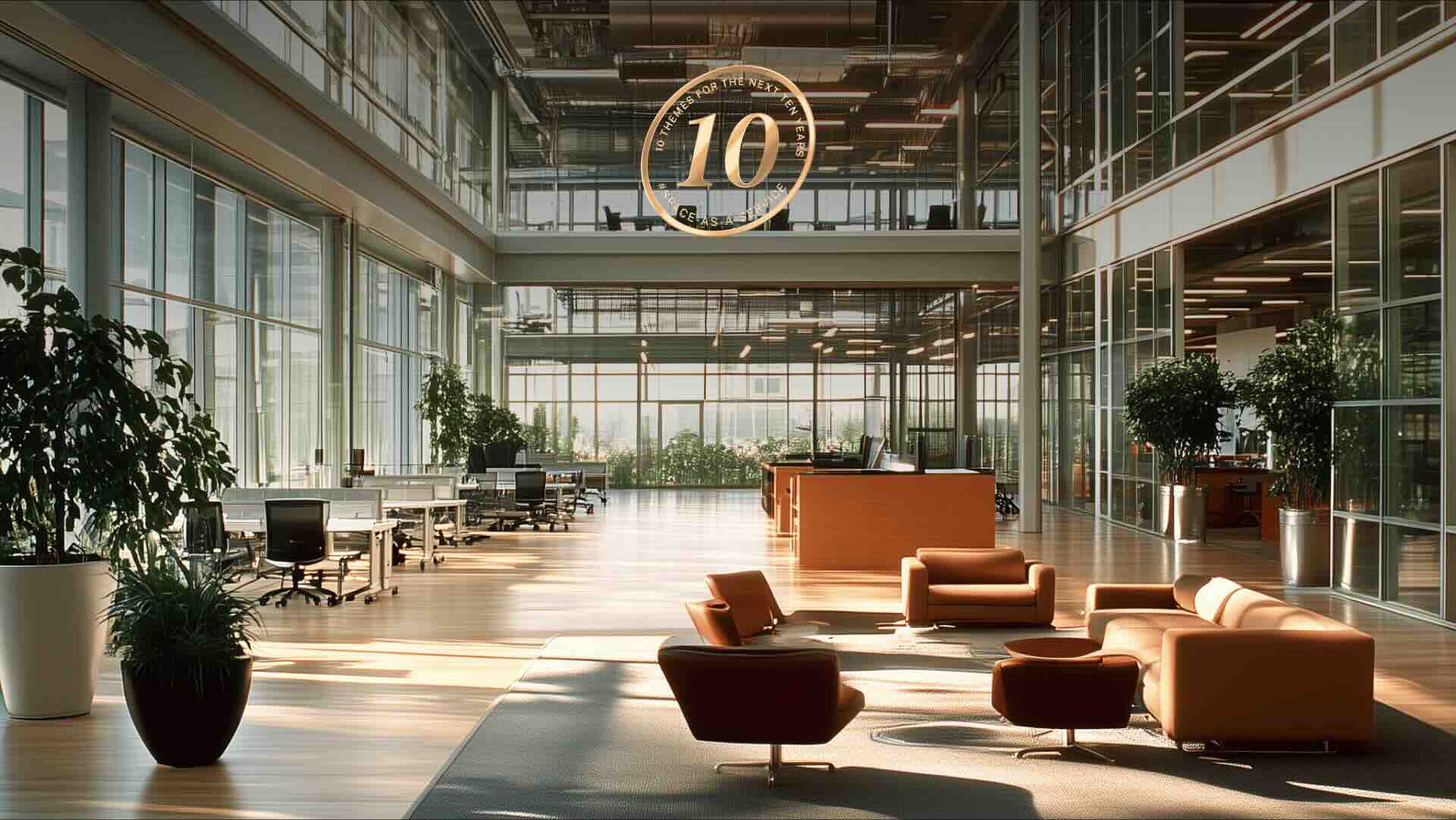
Number 6: Outcomes as a Service - Beyond Software to Solutions
.png)
All you need to know about Nvidia's upcoming AI Supercomputers
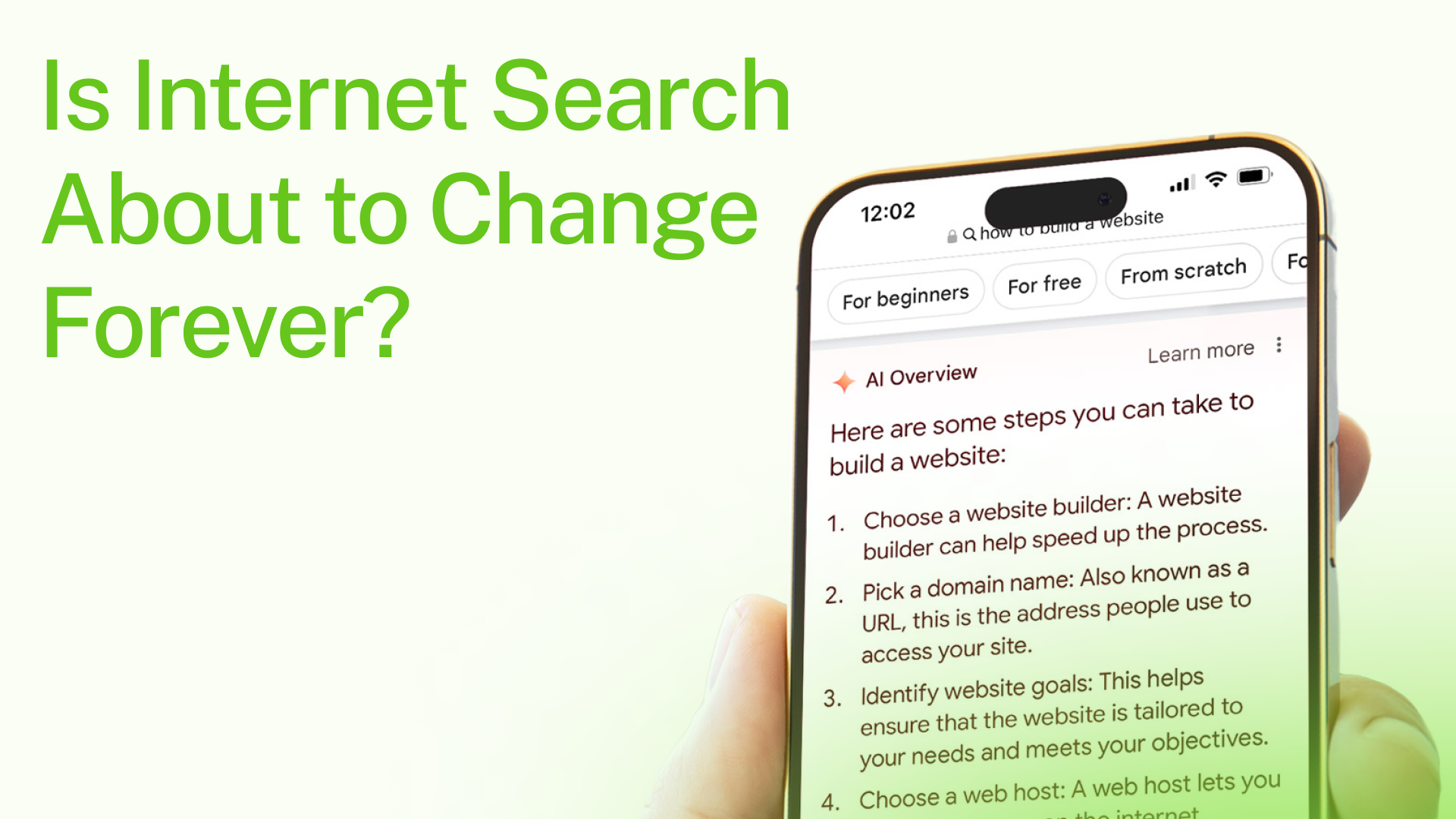
What AI Search Means for the World and the Work
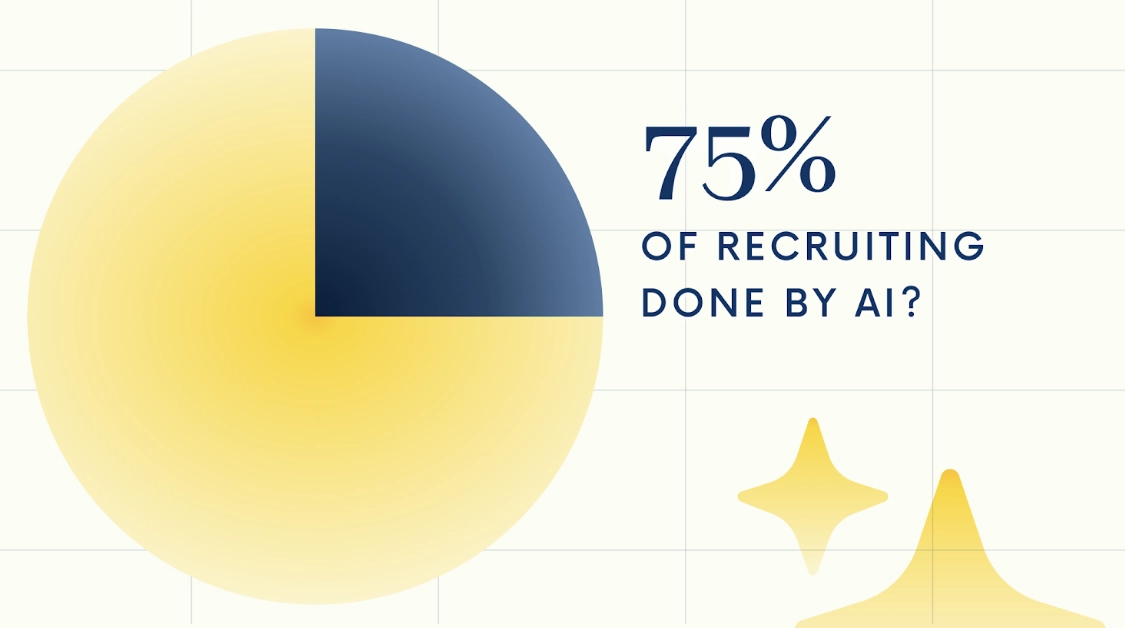
AI in Recruiting: How Did We Get Here?
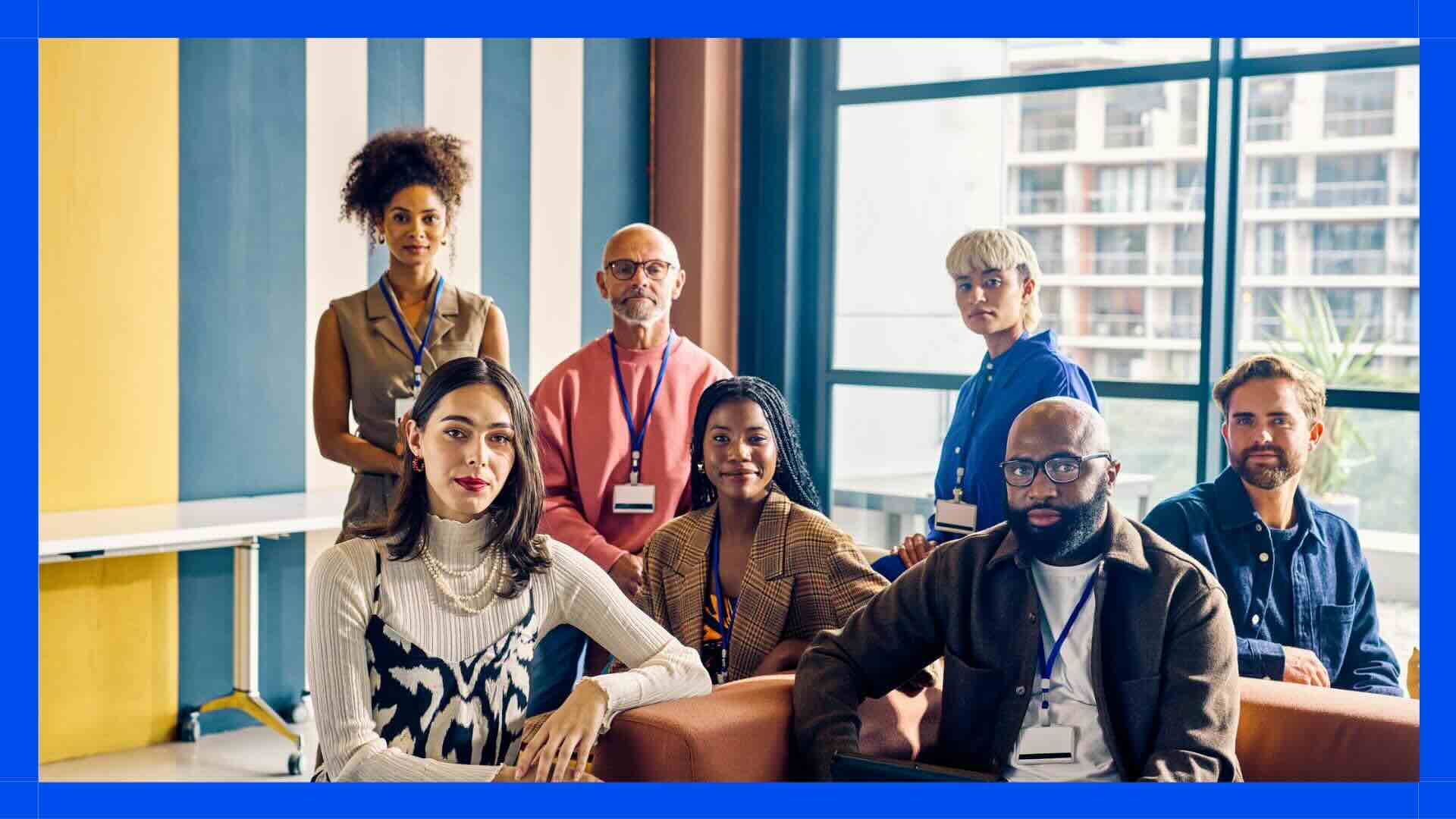